class: center, middle, inverse, title-slide # 4.5 — Bayesian Players ## ECON 316 • Game Theory • Fall 2021 ### Ryan Safner
Assistant Professor of Economics
safner@hood.edu
ryansafner/gameF21
gameF21.classes.ryansafner.com
--- class: inverse # Outline ### [Bayesian Statistics](#3) ### [Bayes’ Rule Example](#19) --- class: inverse, center, middle # Bayesian Statistics --- # Bayesian Statistics .pull-left[ .smallest[ - Most people’s understanding & intuitions of probability are about the **objective** .hi[frequency] of events occurring - “If I flip a fair coin many times, the probability of Heads is 0.50” - “If this election were repeated many times, the probability of Biden winning is 0.60” - This is known as the .hi[“frequentist”] interpretation of probability - And is almost entirely the only thing taught to students (because it’s easier to explain) ] ] .pull-right[ .center[ 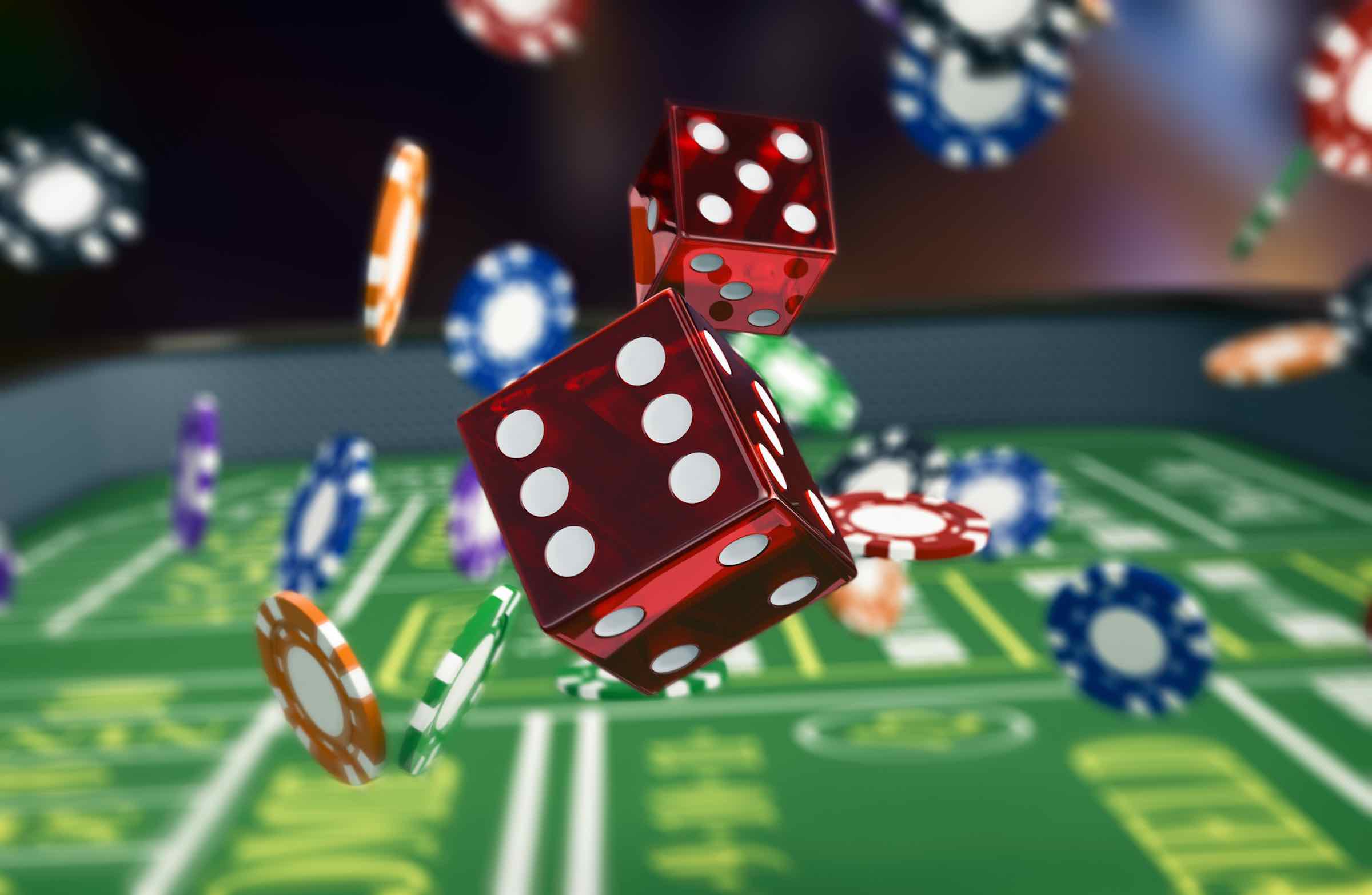 ] ] --- # Bayesian Statistics .pull-left[ .smallest[ - Another valid (competing) interpretation is probability represents our **subjective** .hi[belief] about an event - “I am 50% certain the next coin flip will be Heads” - “I am 60% certain that Biden will win the election” - This is particularly useful for **unique** events (that occur once...and really, isn’t that every event in the real world?) - This is known as the .hi[“Bayesian”] interpretation of probability ] ] .pull-right[ .center[ 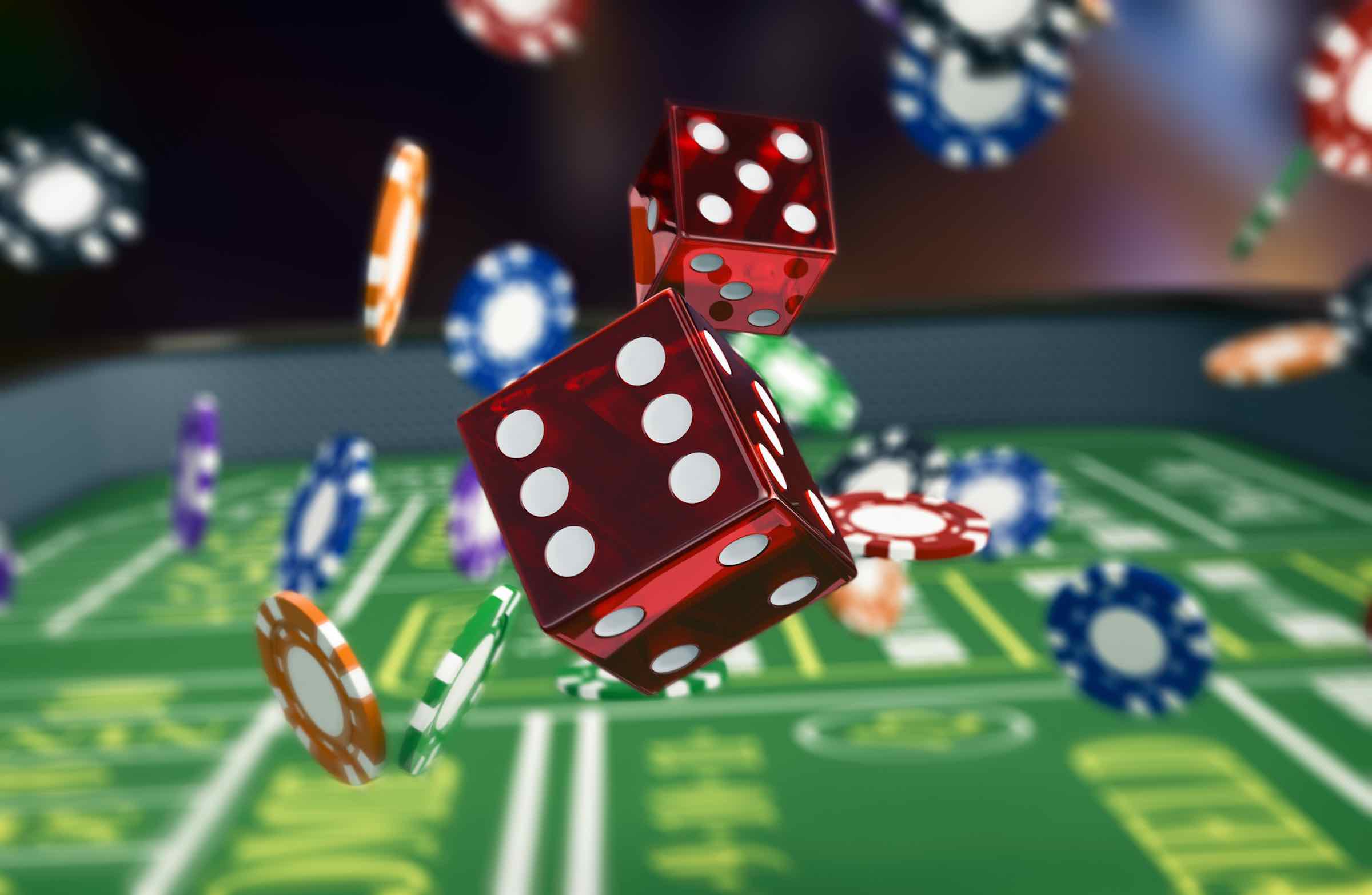 ] ] --- # Bayesian Statistics .pull-left[ - In .hi-purple[Bayesian statistics], probability measures the degree of certainty about an event - Beliefs range from impossible `\((p=0)\)` to certain `\((p=1)\)` - This conditions probability on your **beliefs** about an event ] .pull-right[ .center[ 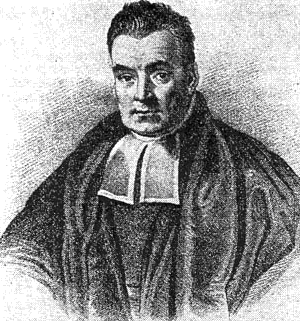 .smallest[ Rev. Thomas Bayes 1702—1761 ] ] ] --- # Bayesian Statistics .pull-left[ .quitesmall[ - The bread and butter of thinking like a Bayesian is .hi-purple[updating your beliefs in response to new evidence] - You have some .hi[prior] belief about something - New evidence should **update** your belief (level of certainty) about it - Updated belief known as your .hi[posterior] belief - Your beliefs are *not* completelydetermined by the latest evidence, new evidence just *slightly* changes your beliefs, proportionate to how compelling the evidence is - .hi-purple[This is fundamental to modern science and having rational beliefs] - And some mathematicians will tell you, the *proper* use of statistics ] ] .pull-right[ .center[ 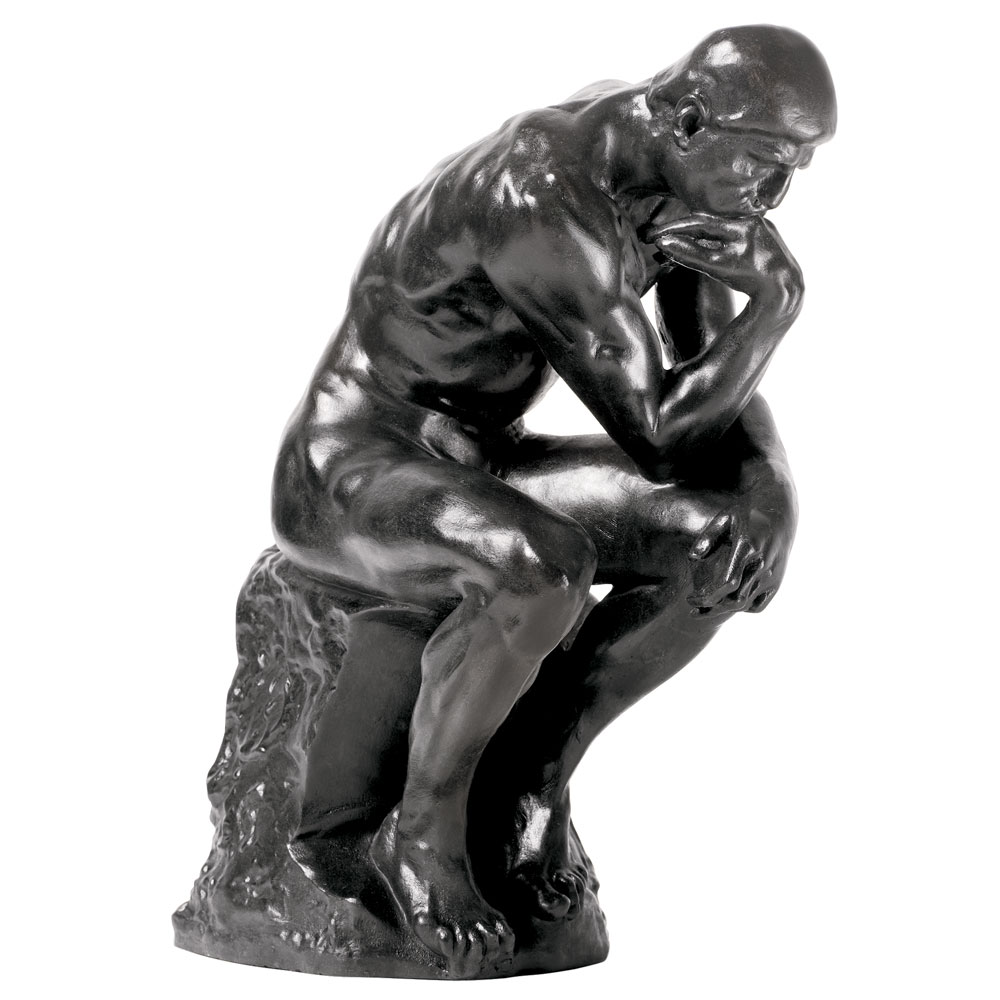 ] ] --- # Bayesian Statistics Examples .pull-left[ .smallest[ 1. You are a bartender. If the next person that walks in is wearing a kilt, what is the probability s/he wants to order Scotch? 2. You are playing poker and the player before you raises. 3. What is the probability that someone has watched the Superbowl? What if you learn that person is a man? 4. You are a policymaker deciding foreign policy, and get a new intelligence report. 5. You are trying to buy a home and make an offer, which the seller declines. ] ] .pull-right[ .center[ 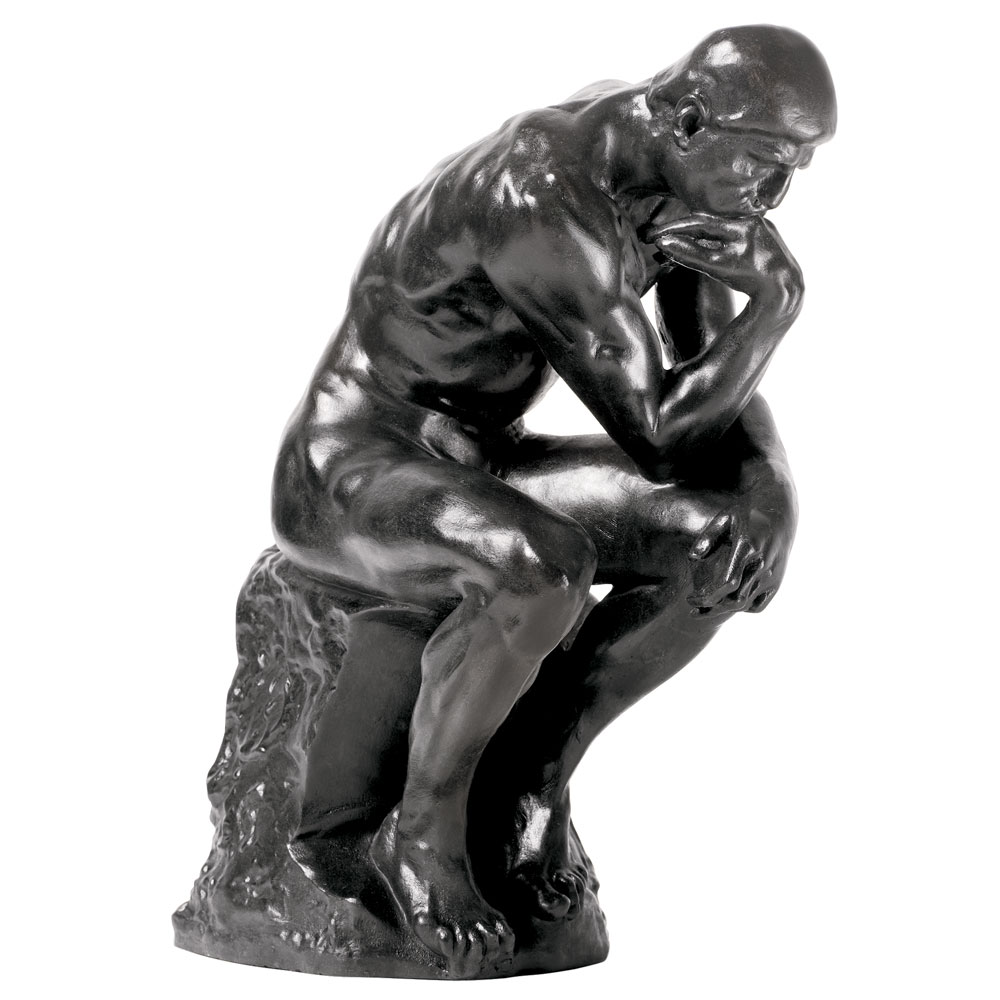 ] ] .pull-right[ .center[ 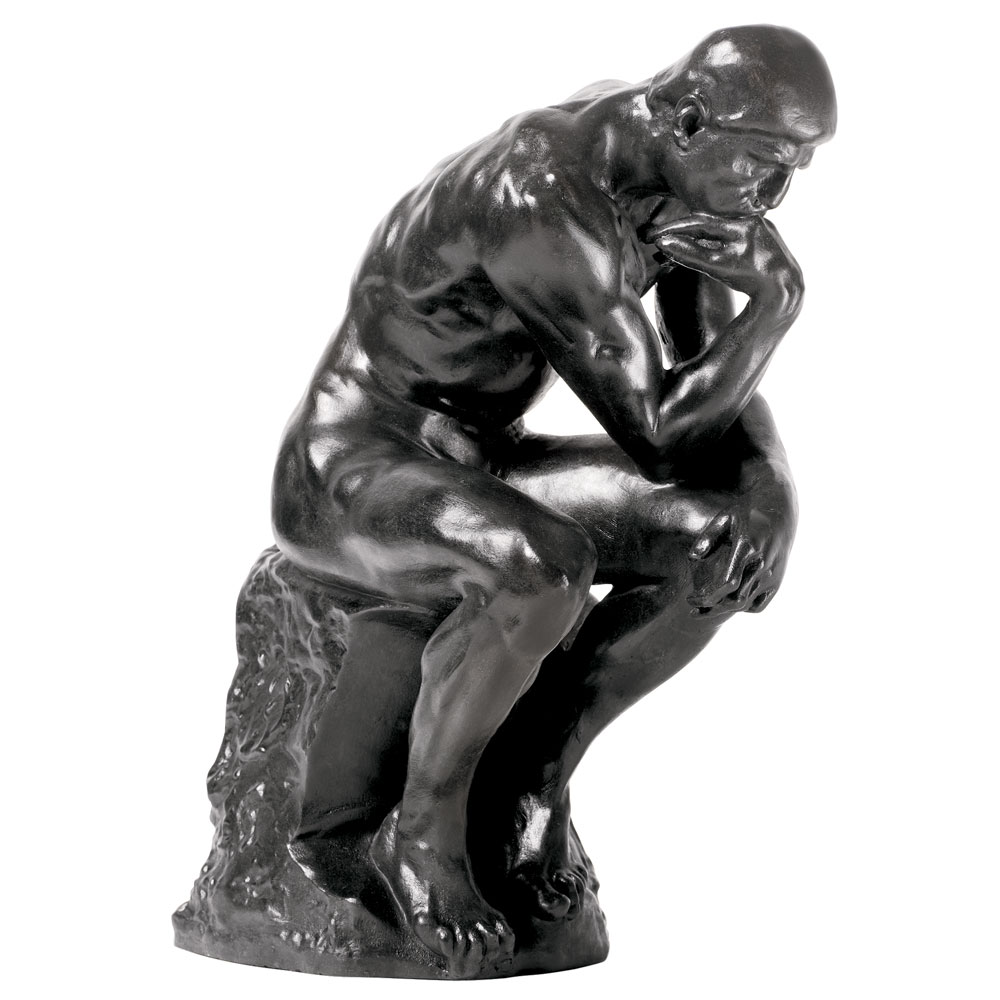 ] ] --- # Conditional Probability .pull-left[ .smallest[ - All of this revolves around .hi-purple[conditional probability]: the probability of some event `\(B\)` occurring, given that event `\(A\)` has already occurred `$$P(B|A) = \frac{P(A \text{ and } B)}{P(A)}$$` - `\(P(B|A)\)`: “Probability of `\(B\)` given `\(A\)`” ] ] .pull-right[ .center[ 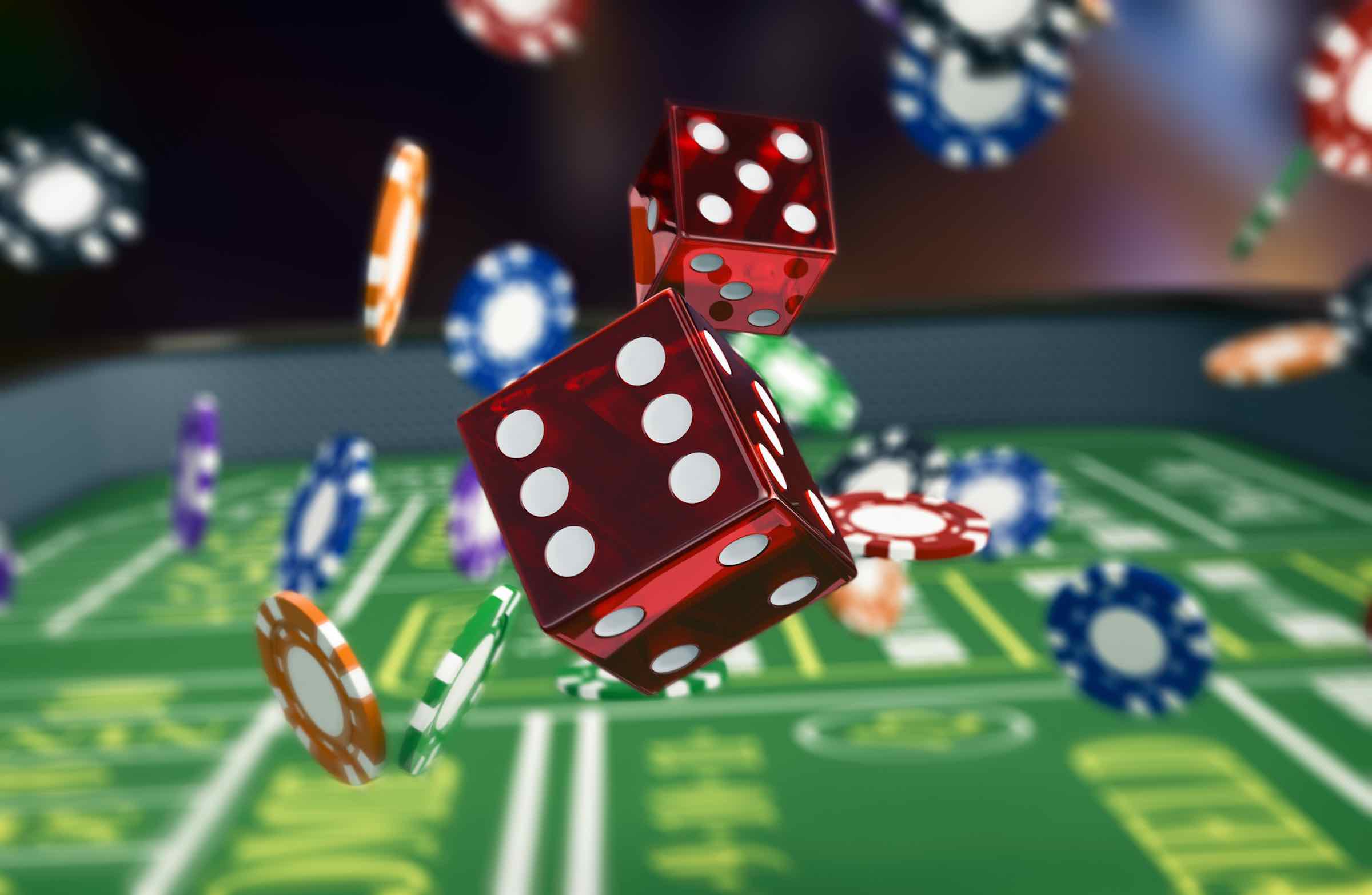 ] ] --- # Conditional Probability .pull-left[ .smallest[ - All of this revolves around .hi-purple[conditional probability]: the probability of some event `\(B\)` occurring, given that event `\(A\)` has already occurred `$$P(B|A) = \frac{P(A \text{ and } B)}{P(A)}$$` - If we know `\(A\)` has occurred, `\(P(A)>0\)`, and then every outcome that is `\(\neg A\)` (“not A”) cannot occur `\((P(\neg A)=0)\)` ] ] .pull-right[ <img src="4.5-slides_files/figure-html/unnamed-chunk-1-1.png" width="504" style="display: block; margin: auto;" /> ] --- # Conditional Probability .pull-left[ .smallest[ - All of this revolves around .hi-purple[conditional probability]: the probability of some event `\(B\)` occurring, given that event `\(A\)` has already occurred `$$P(B|A) = \frac{P(A \text{ and } B)}{P(A)}$$` ] ] .pull-right[ <img src="4.5-slides_files/figure-html/unnamed-chunk-2-1.png" width="504" style="display: block; margin: auto;" /> ] --- # Conditional Probability .pull-left[ .smallest[ - All of this revolves around .hi-purple[conditional probability]: the probability of some event `\(B\)` occurring, given that event `\(A\)` has already occurred `$$P(B|A) = \frac{P(A \text{ and } B)}{P(A)}$$` - If we know `\(A\)` has occurred, `\(P(A)>0\)`, and then every outcome that is `\(\neg A\)` (“not A”) cannot occur `\((P(\neg A)=0)\)` - The only part of `\(B\)` which can occur if `\(A\)` has occurred is `\(A\)` and `\(B\)` - Since the sample space `\(S\)` must equal 1, we’ve reduced the sample space to `\(A\)`, so we must rescale by `\(\frac{1}{P(A)}\)` ] ] .pull-right[ <img src="4.5-slides_files/figure-html/unnamed-chunk-3-1.png" width="504" style="display: block; margin: auto;" /> ] --- # Conditional Probability .pull-left[ `$$P(B|A) = \frac{P(A \text{ and } B)}{P(A)}$$` - If events `\(A\)` and `\(B\)` were .hi-turquoise[independent], then the probability `\(P(A\)` and `\(B)\)` happening would be just `\(P(A) \times P(B)\)` - `\(P(A|B) = P(A)\)` - `\(P(B|A) = P(B)\)` ] .pull-right[ <img src="4.5-slides_files/figure-html/unnamed-chunk-4-1.png" width="504" style="display: block; margin: auto;" /> ] --- # Conditional Probability .pull-left[ `$$P(B|A) = \frac{P(A \text{ and } B)}{P(A)}$$` - But if they are *not* independent, it’s `\(P(A \text{ and } B) = P(A) \times P(B|A)\)` - (Just multiplying both sides above by the denominator, `\(P(A))\)` ] .pull-right[ <img src="4.5-slides_files/figure-html/unnamed-chunk-5-1.png" width="504" style="display: block; margin: auto;" /> ] --- # Conditional Probability and Bayes’ Rule .pull-left[ .smallest[ - Bayes realized that the conditional probabilities of two non-independent events are proportionately related `$$\color{green}{P(B|A)} = \frac{P(A \text{ and } B)}{\color{red}{P(A)}}$$` `$$\color{orange}{P(A|B)} = \frac{P(A \text{ and } B)}{\color{blue}{P(B)}}$$` ] ] .pull-right[ <img src="4.5-slides_files/figure-html/unnamed-chunk-6-1.png" width="504" style="display: block; margin: auto;" /> ] --- # Conditional Probability and Bayes’ Rule .pull-left[ .smallest[ - Bayes realized that the conditional probabilities of two non-independent events are proportionately related `$$\color{green}{P(B|A)} = \frac{P(A \text{ and } B)}{\color{red}{P(A)}}$$` `$$\color{orange}{P(A|B)} = \frac{P(A \text{ and } B)}{\color{blue}{P(B)}}$$` `$$\color{orange}{P(A|B)}\color{blue}{P(B)} = P(A \text{ and }B) = \color{green}{P(B|A)}\color{red}{P(A)}$$` ]] .pull-right[ <img src="4.5-slides_files/figure-html/unnamed-chunk-7-1.png" width="504" style="display: block; margin: auto;" /> ] --- # Conditional Probability and Bayes’ Rule .pull-left[ .smallest[ - Bayes realized that the conditional probabilities of two non-independent events are proportionately related `$$\color{green}{P(B|A)} = \frac{P(A \text{ and } B)}{\color{red}{P(A)}}$$` `$$\color{orange}{P(A|B)} = \frac{P(A \text{ and } B)}{\color{blue}{P(B)}}$$` `$$\color{orange}{P(A|B)}\color{blue}{P(B)} = P(A \text{ and }B) = \color{green}{P(B|A)}\color{red}{P(A)}$$` - Divide everything by .blue[P(B)], you get, famously, .hi[Bayes’ rule]: `$$\color{orange}{P(A|B)} = \frac{\color{green}{P(B|A)}\color{red}{P(A)}}{\color{blue}{P(B)}}$$` ] ] .pull-right[ <img src="4.5-slides_files/figure-html/unnamed-chunk-8-1.png" width="504" style="display: block; margin: auto;" /> ] --- # Bayes’ Rule: Hypotheses and Evidence .pull-left[ .smallest[ - The `\(A\)`’s and `\(B\)`’s are rather difficult to remember if you don’t use this often - A lot of people prefer to think of .hi[Bayes’ rule] in terms of a hypothesis you have `\((H)\)`, and new evidence or data `\(e\)` `$$P(H|e) = \frac{P(e|H)p(H)}{P(e)}$$` - `\(P(H|e)\)`: .hi-purple[posterior ] your hypothesis is correct given the new evidence - `\(P(e|H)\)`: .hi-purple[likelihood] of seeing the evidence under your hypothesis - `\(P(H)\)`: .hi-purple[prior belief] in of your hypothesis - `\(P(e)\)`: .hi-purple[average likelihood] of seeing the evidence under *any/all* hypothesis ] ] .pull-right[ .center[ 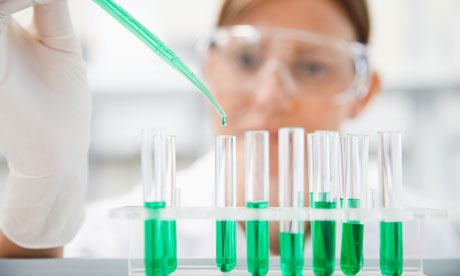 ] ] --- class: inverse, center, middle # Bayes’ Rule Example --- # Bayes’ Rule Example .pull-left[ .quitesmall[ .content-box-green[ .hi-green[Example]: Suppose 1% of the population has a rare disease. A test that can diagnose the disease is 95% accurate. What is the probability that a person who takes the test and comes back positive has the disease? ] - What would you guess the probability is? ] ] .pull-right[ .center[ 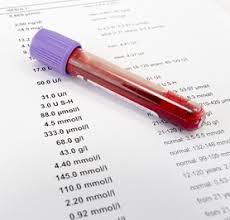 ] ] --- # Bayes’ Rule Example .pull-left[ .quitesmall[ .content-box-green[ .hi-green[Example]: Suppose 1% of the population has a rare disease. A test that can diagnose the disease is 95% accurate. What is the probability that a person who takes the test and comes back positive has the disease? ] ] .quitesmall[ - `\(P(\text{Disease}) = 0.01\)` - `\(P(+|\text{Disease}) =0.95 = P(-|\neg \text{Disease})\)` - We know `\(P(+|\text{Disease})\)` but want to know `\(P(\text{Disease}|+)\)` - **These are not the same thing!** - Related by Bayes’ Rule: `$$P(\text{Disease}|+)=\frac{P(+|\text{Disease})P(\text{Disease})}{P(+)}$$` ] ] .pull-right[ .center[ 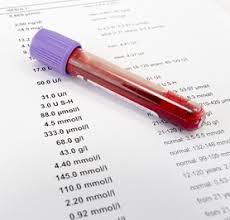 ] ] --- # Bayes’ Rule Example .pull-left[ - `\(P(\text{Disease}) = 0.01\)` - `\(P(+|\text{Disease}) =0.95 = P(-|\neg \text{Disease})\)` `$$P(\text{Disease}|+)=\frac{P(+|\text{Disease})P(\text{Disease})}{P(+)}$$` - What is `\(P(+)\)`?? ] .pull-right[ .center[ 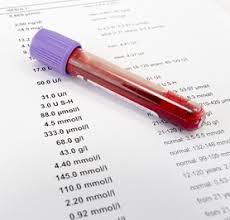 ] ] --- # Bayes’ Rule Example .pull-left[ - What is the total probability of `\(B\)` in the diagram? `$$\begin{align*}P(B)&=P(B \text{ and } A)+P(B \text{ and } \neg A)\\ &=P(B|A)P(A)+P(B|\neg A)P(\neg A) \\ \end{align*}$$` - This is known as the law of total probability ] .pull-right[ <img src="4.5-slides_files/figure-html/unnamed-chunk-9-1.png" width="504" style="display: block; margin: auto;" /> ] --- # Bayes’ Rule Example: Aside .pull-left[ - Because we usually have to figure out `\(P(B)\)` (the denominator), Bayes’ rule is often expanded to `$$P(B|A)=\frac{P(A|B)P(A)}{P(B|A)P(A)+P(B|\neg A)P(\neg A)}$$` - Assuming there are two possibilities `\((A\)` and `\(\neg A)\)`, e.g. True or False ] .pull-right[ <img src="4.5-slides_files/figure-html/unnamed-chunk-10-1.png" width="504" style="display: block; margin: auto;" /> ] --- # Bayes’ Rule Example: Aside .pull-left[ - If there are more than two possibilities, you can further expand it to `\(\displaystyle\sum^n_{i=1}P(B|A_i)P(A_i)\)` for `\(n\)` number of possible alternatives to `\(A\)` ] .pull-right[ <img src="4.5-slides_files/figure-html/unnamed-chunk-11-1.png" width="504" style="display: block; margin: auto;" /> ] --- # Bayes’ Rule Example .pull-left[ .smallest[ - What is the total probability of `\(+\)`? ] .quitesmall[ `$$\begin{align*}P(+)&=P(+ \text{ and Disease})+P(+ \text{ and } \neg \text{ Disease})\\ &=P(+|\text{Disease})P(\text{Disease})+P(+|\neg \text{Disease})P(\neg \text{Disease}) \\ \end{align*}$$` ] .smallest[ - `\(P(\text{Disease}) = 0.01\)` - `\(P(+|\text{Disease}) =0.95\)` ] ] .pull-right[ <img src="4.5-slides_files/figure-html/unnamed-chunk-12-1.png" width="504" style="display: block; margin: auto;" /> ] --- # Bayes’ Rule Example .pull-left[ .smallest[ - What is the total probability of `\(+\)`? ] .quitesmall[ `$$\begin{align*}P(+)&=P(+ \text{ and Disease})+P(+ \text{ and } \neg \text{ Disease})\\ &=P(+|\text{Disease})P(\text{Disease})+P(+|\neg \text{Disease})P(\neg \text{Disease}) \\ \end{align*}$$` ] .smallest[ - `\(P(\text{Disease}) = 0.01\)` - `\(P(+|\text{Disease}) =0.95\)` `$$P(+)=0.95(0.01)+0.05(0.99)=0.0590$$` ] ] .pull-right[ <img src="4.5-slides_files/figure-html/unnamed-chunk-13-1.png" width="504" style="display: block; margin: auto;" /> ] --- # Bayes’ Rule Example .pull-left[ | | Disease | `\(\neg\)` Disease | Total | |----|---------|----------------|-------| | + | 0.0095 | 0.0495 | **0.0590** | | - | 0.0005 | 0.9405 | 0.9410 | | Total | 0.0100 | 0.9900 | 1.0000 | ] .pull-right[ .center[ 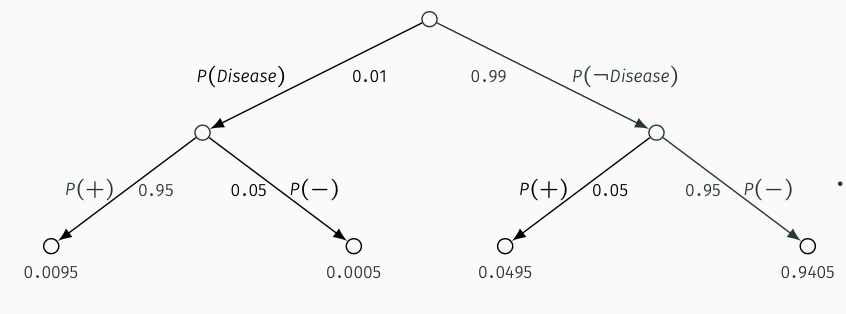 ] ] --- # Bayes’ Rule Example .pull-left[ .smallest[ - `\(P(\text{Disease}) = 0.01\)` - `\(P(+|\text{Disease}) =0.95 = P(-|\neg \text{Disease})=0.95\)` - `\(P(+)=0.0590\)` ] ] .pull-right[ .center[ 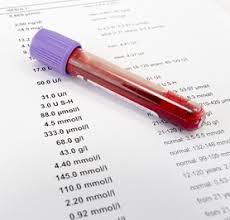 ] ] --- # Bayes’ Rule Example .pull-left[ .smallest[ - `\(P(\text{Disease}) = 0.01\)` - `\(P(+|\text{Disease}) =0.95 = P(-|\neg \text{Disease})=0.95\)` - `\(P(+)=0.0590\)` `$$\begin{align*}P(\text{Disease}|+)&=\frac{P(+|\text{Disease})P(\text{Disease})}{P(+)}\\ \end{align*}$$` ] ] .pull-right[ .center[ 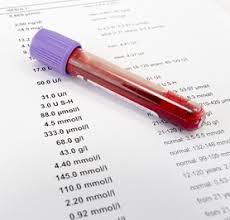 ] ] --- # Bayes’ Rule Example .pull-left[ .smallest[ - `\(P(\text{Disease}) = 0.01\)` - `\(P(+|\text{Disease}) =0.95 = P(-|\neg \text{Disease})=0.95\)` - `\(P(+)=0.0590\)` `$$\begin{align*}P(\text{Disease}|+)&=\frac{P(+|\text{Disease})P(\text{Disease})}{P(+)}\\ P(\text{Disease}|+)&=\frac{0.95 \times 0.01}{0.0590}\\ &= 0.16 \\\end{align*}$$` - The probability you have the disease is only 16%! - Most people vastly overestimate because they forget the base rate of the disease, `\(P(\text{Disease})\)` is so low (1%)! ] ] .pull-right[ .center[ 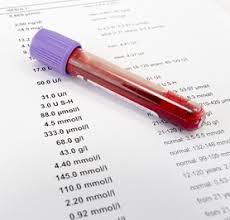 ] ] --- # Bayes’ Rule and Bayesian Updating .pull-left[ - Bayes Rule tells us how we should update our beliefs given new evidence .center[ 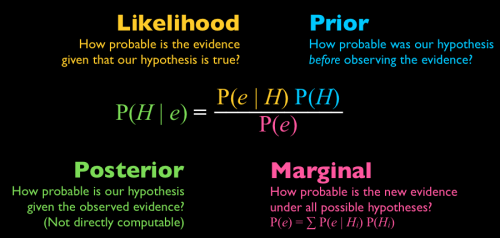 ] ] .pull-right[ .center[ 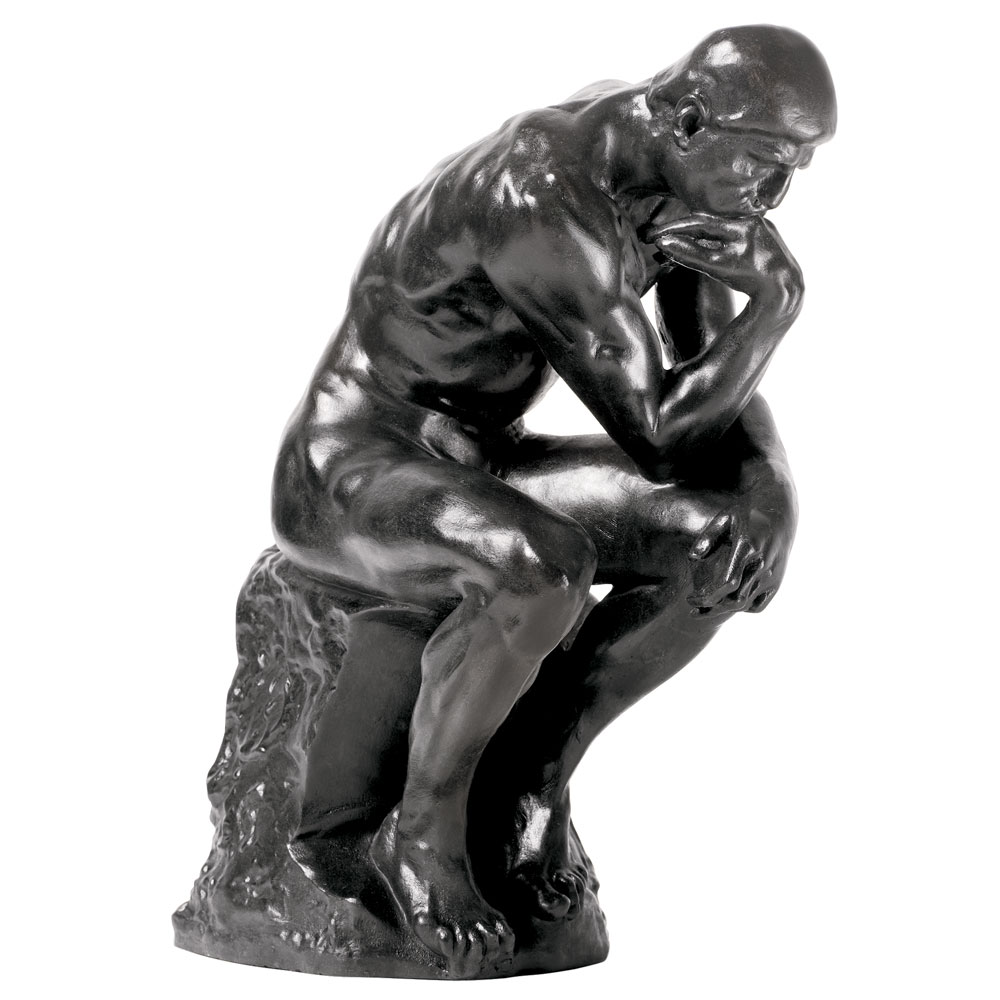 ] ] --- # Highly, Highly Recommended .center[ <iframe width="980" height="550" src="https://www.youtube.com/embed/HZGCoVF3YvM" title="YouTube video player" frameborder="0" allow="accelerometer; autoplay; clipboard-write; encrypted-media; gyroscope; picture-in-picture" allowfullscreen></iframe> ] --- # Highly, Highly Recommended .center[ <iframe width="980" height="550" src="https://www.youtube.com/embed/lG4VkPoG3ko" title="YouTube video player" frameborder="0" allow="accelerometer; autoplay; clipboard-write; encrypted-media; gyroscope; picture-in-picture" allowfullscreen></iframe> ]